
We argue that size curve forecasting, also called size break or size split forecasting, is a forsaken part of inventory planning that has the potential to make or break your sales targets. It is the practice of determining an optimal size distribution across your products based on their true demand. If you want to meet your sales targets and keep lean but sufficient stock levels, ordering and carrying the right sizes across your assortment is crucial.
We are not breaking any news when we say that the size curve is neglected. In fact, research has shown that a forecast error for size curves commonly ranges up to 40% for many items in the apparel industry, and so it has been for a long time. In this article, we take a closer look at what that means in practice and actual numbers.
The hardships of size curve forecasting
If it’s not news, why is size curve forecasting such a long-standing issue?
When it comes to inventory planning and stock optimization brands are widely aware that the accuracy of their purchase decisions is a determining factor of their ability to meet sales goals and keep a healthy cash flow. Demand forecasting is however notoriously hard and many brands struggle to get it right.
Even within their best-selling product categories, many brands are struggling with overstock in specific sizes and stockouts in others. If sales forecasting for a certain product is hard, getting it right on SKU level is almost impossible for most brands. MOQs not making it any easier. However, it is pivotal for accurate demand and inventory planning.
Common pitfalls of size curve forecasting
Attempting to forecast a size curve, some brands look at sales history as a basis for size forecasts and some brands simply refer to the recommendations of their suppliers or order an average curve of the most common sizes. Many simply base the entirety of their inventory purchases on last year’s sales and their projected year-on-year growth.
Even when actively forecasting demand for specific sizes it’s a cumbersome task when done manually. It is prone to human errors and is often largely based on intuition and incomplete data. Brands that do it usually base their orders for replenishment or new product launches on historical sales data. Even though a great practice, what they often fail to take into consideration is historic stockouts. What if the product you are basing your forecasts on was out of stock for a period of time? If you are not taking missed sales into consideration when forecasting a new size cure, you are bound to repeat the same stockouts.
All of these scenarios leave brands with unwanted overstock and missed sales due to faulty size curves across their entire assortment. And so, miscalculating or neglecting the size curve has a potentially large effect on a brand’s cash flow.
Let's look at some examples:
Company A
Company A is placing a new order for summer. Included in that order is a batch of Shirt X.
They estimate that they need to buy 100 of Shirt X to meet their sales projections. As they always do, they order a standard split of sizes, a so-called “normal distribution”, for those 100 shirts. This looks like follows:
Say that the total number of shirts, 100, is indeed an accurate forecast. However, the estimated true size demand (actual demand) and the result (realized sales) look more like this:
It’s easy to gather that this means that Company A has missed sales on sizes S, M, and L but is sitting on unwanted overstock on sizes XS and XL. Shirt X retails at € 70, which means a loss of € 1344 in just missed sales.
The overstock comes with further costs for handling, marketing, and loss of margins if they end up selling the shirts at a discounted price down the line (this is to say if they manage to sell them at all).
The full picture, taking purchase price, vat, and margins into consideration, reveals this:
Result
▸ Sell-through: 76%
▸ Revenue excl VAT: € 4 256
▸ Margin value: € 2 736
▸ Loss of revenue: € 1 344 (24%)
▸ Loss of margin: € 864 (24%)
Potential inventory error
▸ Stock value: € 480 (24% of purchase)

Company B
Company B makes a point of always forecasting down to SKU level when planning new purchase orders. They take historic sales into consideration when estimating what to buy for the next sales period (6 months). The purchase estimate looks like this:
They look at the last period sales split to guide their new order:
What Company B fails to take into consideration is that during the last period, there were multiple stockouts in some of the sizes, now skewing their new forecast. Looking at size S, the new purchase estimates based on last period sales would result in this:
The 6 month sales period with deliveries month 1, 3 and 5.
Result
▸ Potential loss in sales: € 91 000
▸ Loss in % of total purchase: 16%
▸ Loss of sales in size S: 54%
During the sales period, size S was out of stock two times waiting for the next delivery. Meaning they missed out on potential sales before the next replenishment. An estimate of the true demand for size S reveals a potential total loss of 54% for size S and 16% in total for that product.

What we can see from Company B’s error is that if historic stockouts are not taken into account, our calculations would be based on the assumption that no products sold equals no demand whilst, in reality, it is due to stockouts. Basing our new purchases on these data, we end up with poor supply again.
The implications of the error-prone process of size curve forecasting
The above examples are simplified with size ranges of just five levels. Oftentimes size ranges are much larger, complicating the forecasting process even more and severely implicating a brand's ability to meet customer demand. A forecast of 1-2% for each size in a curve from XS to XXL could cause an overall error of 10%. Being multiplied across all styles and colors of one single item or the full assortment leads to large errors in the overall inventory.
As we can see from the examples, both Company A and Company B are looking at missed sales and a high risk of overstocking, despite their best efforts. In these articles, you can learn more about the implications of stockouts and overstock.
These examples reveal what not to do as well as what to do to get your size curve right. But let’s break it down for good measure.
How to: size curve forecasting
As we discussed above, when forecasting your size curve it is good practice to look at your historical sales and to take your historical stockouts into account. But what if you are placing an order for a completely new product with no sales data or a product with very little historical data? And what if the product does have those historical stockouts we keep going on about, how do you actually account for them?
First off, it is worth mentioning that the size curve is more constant in distribution over time than an overall demand forecast for a product. Demand will vary based on season, economic climates, and due to campaigns. The size curve will however remain similarly proportioned over time even as overall demand ebbs and flows. This means you can reliably calculate the size curve of a new pair of jeans by looking at your usual size distribution in a similar product, for example, other colors of the same model. This makes it possible to compensate for the lack of data for one product at a given time by utilizing data from similar products.
Of course, the size curve distribution can also vary somewhat over time as different fits go in and out of fashion (e.g. oversized fit, slim fit). Adding new sizes to your range will also introduce skews and challenges in your size curve forecast. However, though a size curve distribution varies over time it is still not as volatile as overall shifts in demand.
With that said, here is a recap of what you need to be able to calculate your size curve:
Give way to statistics over intuition
All hale to intuition, it is an important tool and you will always be sitting on insights that data can’t show you. However, it is important to move away from the guessing game and to start utilizing data to make informed decisions whether it be in excel or forecasting software.Ensuring reliable datasets
Make sure you are using a statistically reliable dataset for your forecasts. As mentioned above, you need enough data on a given variant (style and color) to generate a reliable forecast for your sizes. If you do not have that, opt to use a similar variant or product to enrich the dataset. It will give you a better idea of your size curve.Compensating for historical stockouts
If possible, disqualify that period and use data from a long enough period when the product was in stock. If you do not have that, again use a similar variant or product to enrich the data set. It’s critical to choose products with as many common attributes as possible to the product you are forecasting for.The Bayesian approach
If manually calculating your size curve, this approach allows you to combine different sources of data in your calculations. It makes it possible to calculate your size curve by using data from different samples (to avoid periods of stockouts and promotions).Account for current stock and placed orders
Once you have your size curve forecast, account for current stock levels and potential incoming orders when drafting your new purchase order.
A planning software replaces the rules of thumb and guessing games with a scientifically generated granular forecast for inventory planning and purchasing. Scientifically-driven estimating techniques help you minimize human error and provide repeatable forecasting improvements that become more accurate and more efficient over time.
Forecast size curves in Madden Analytics
Madden Analytics provides you with multiple AI forecasting models optimized for different types of products. You can forecast in bulk and down to SKU level. When drafting your forecasts and purchase orders you can see the recommended size curve generated for each product based on projected sales.
The recommended size curve is based on your historical and current sales and compensates for eventual historical stockouts. The algorithm will automatically enrich your data set with relevant data from other variants or products if the data is too scarce.
The overall forecast and size split recommendation takes into account what you already have in stock, what you have already ordered and gives you a forecast based on what you need to order to meet demand.
All forecasts and purchase orders are easy to tweak based on the knowledge and insights of the user, meaning you can adjust the suggested quantities based on knowledge of upcoming campaigns or other variables that could further impact sales or an optimal inventory distribution.
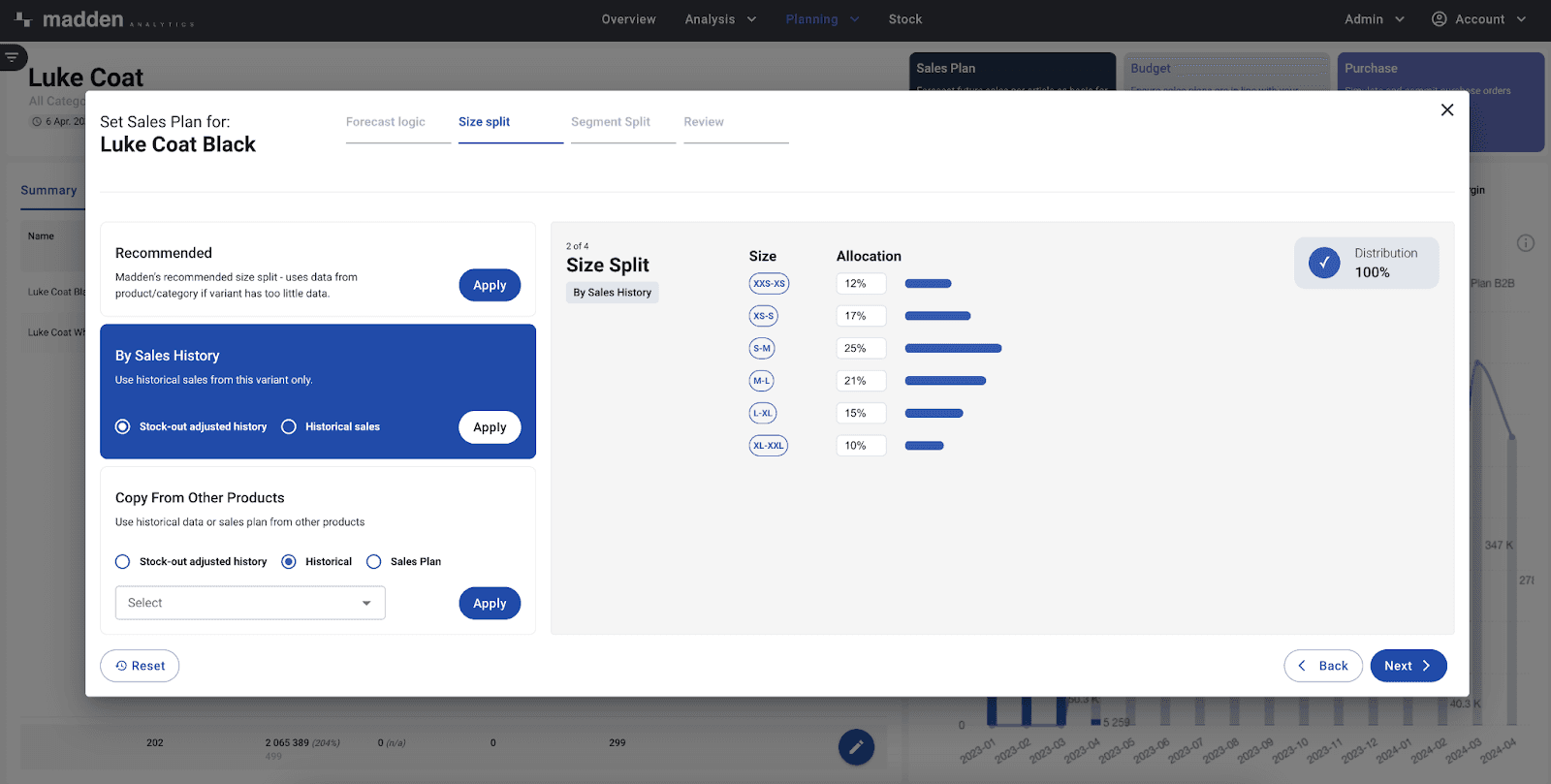
Size curve forecasting is a cumbersome and error-prone endeavor - although with your while. Madden Analytics can help automate this process for you based on reliable data and analytics. If you want to learn more about how Madden Analytics can help you optimize your inventory levels to boost sales and avoid overstock - get in touch for a free demo!
More news
Granit Funktion & Förvaring Partners with Madden Analytics to Drive Data-Driven Inventory Planning and Profitability
Joe Nimble Teams Up with Madden Analytics to Enhance Inventory Planning via Xentral Integration
Corro Chooses Madden Analytics to Elevate Inventory Planning with Seamless Shopify Integration
Newhouse Partners with Madden Analytics to Enhance Inventory Planning through Seamless Business Central Integration by NaviPro
Understatement Implements Madden Analytics to Optimize Multi-Market Inventory Management and Fuel Global Expansion
Subscribe
Get the latest articles on sales and inventory health straight into your inbox.
Get to know Madden